1. Introduction
In the field of clinical research, the pursuit of groundbreaking discoveries and life-saving treatments is often hindered by complex workflows and data management challenges. The sheer volume and diversity of data generated during clinical trials, coupled with the need for seamless collaboration among researchers, pose significant obstacles to efficient and effective research processes. From patient recruitment and data collection to analysis and reporting, each stage of the clinical research workflow demands robust data management solutions. Data lakes – a revolutionary approach to storing, processing, and analyzing vast amounts of structured and unstructured data, is thus critical for efficient data management for clinical research. By harnessing the power of data lakes, clinical researchers can unlock the full potential of their data, streamline their workflows, and accelerate the pace of scientific discovery. In this blog post, we will explore the critical role that data lakes play in enabling seamless clinical research workflows, and how they are transforming the landscape of medical research.
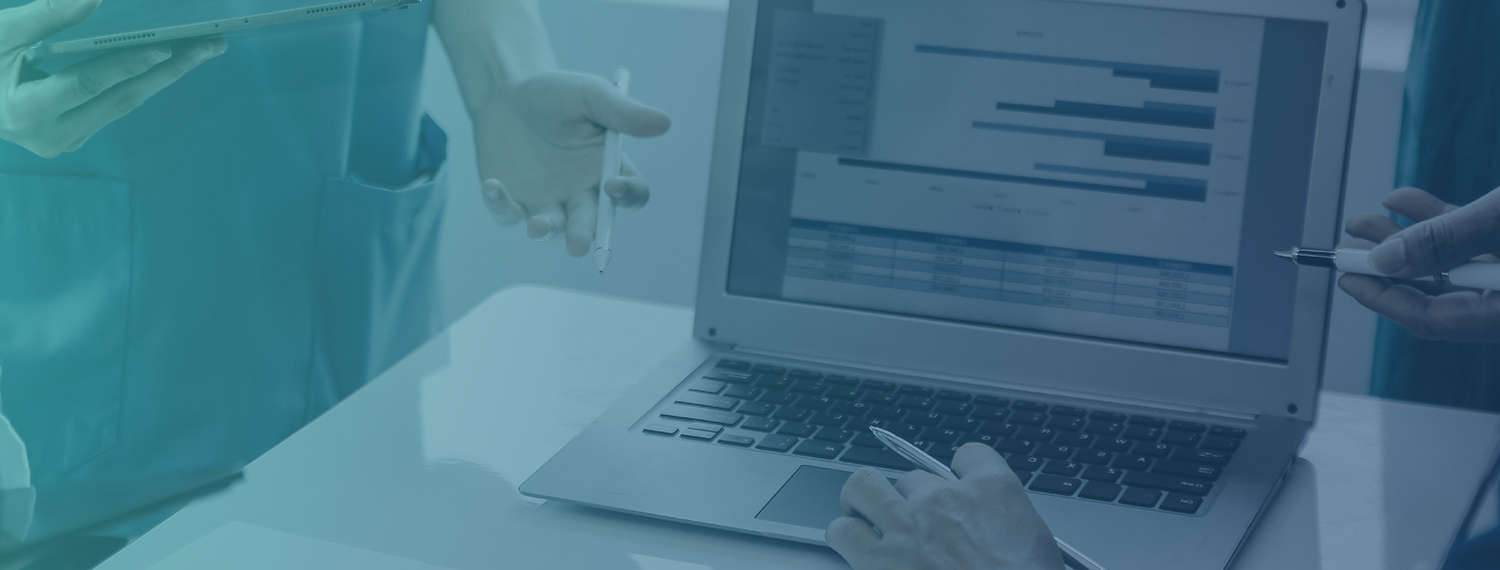
2. Centralization of Data from Disparate Sources
In clinical research, managing data from various sources can be a daunting task. Researchers often encounter challenges such as inconsistent data formats, incompatible systems, and scattered data storage. These issues lead to inefficiencies, delays, and potential errors in the research process. However, the implementation of a data lake can significantly alleviate these challenges by centralizing data from disparate sources. A data lake is a centralized repository that allows for the storage of structured and unstructured data at any scale. By centralizing data in a data lake, researchers can enjoy improved data accessibility, as all relevant information is stored in a single location. This eliminates the need to navigate through multiple systems or databases to retrieve the necessary data. Additionally, data lakes help reduce data silos, which occur when data is isolated and not easily shareable across different departments or teams. With a centralized data lake, researchers can break down these silos and promote collaboration and knowledge sharing. Furthermore, data lakes enhance data integration by enabling the seamless combination of data from various sources. This integration allows for a more comprehensive analysis of the data, leading to deeper insights and better-informed decision-making in clinical research.
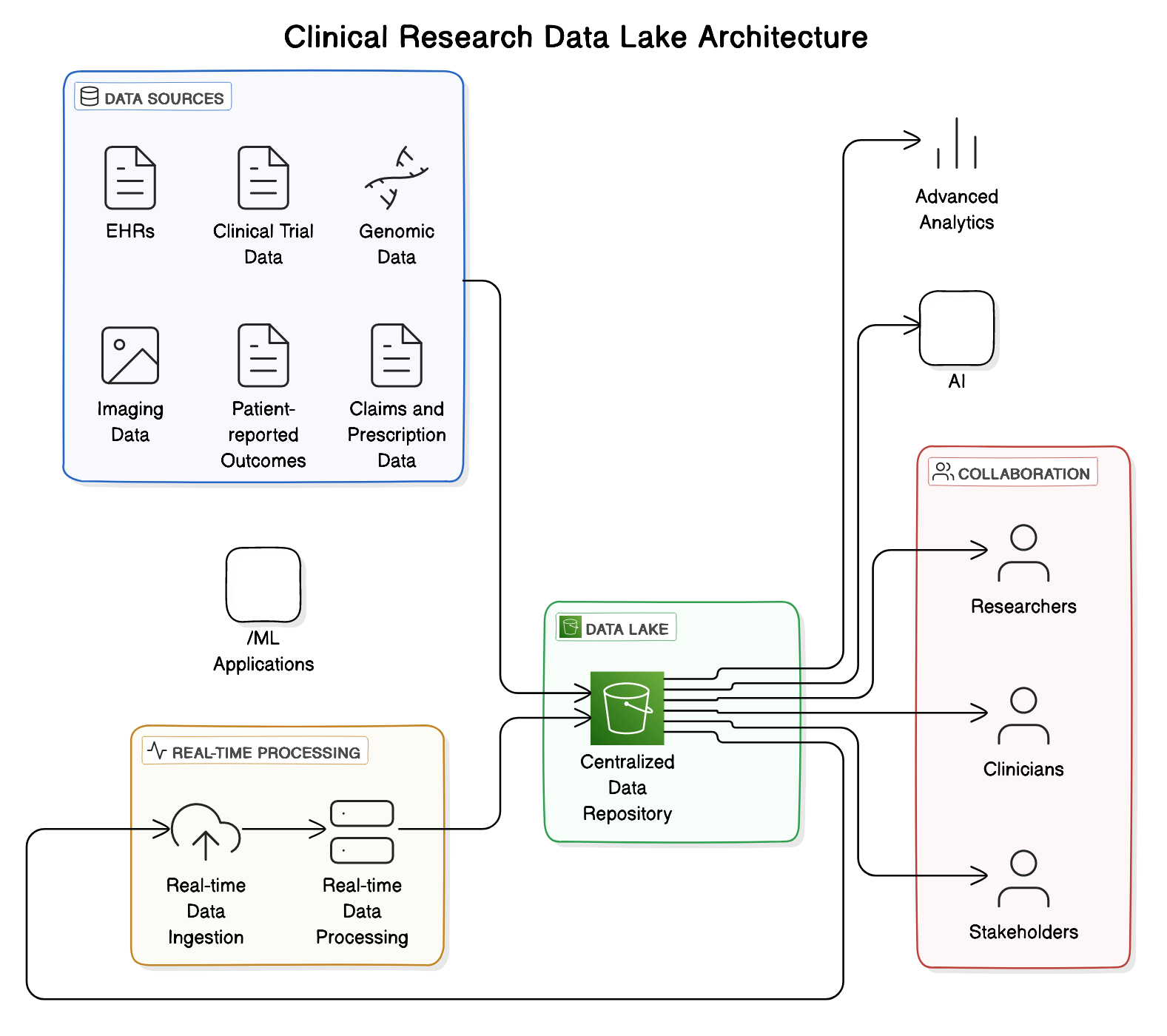
3. Enabling Easy Access and Analysis
A data lake's architecture is designed to facilitate easy access and analysis of data for clinical researchers. By storing data in its raw, unprocessed form, a data lake allows researchers to explore and analyze data without the need for extensive data transformation or preparation. This enables researchers to quickly access and analyze large volumes of data from various sources, such as electronic health records, clinical trial data, and genomic data.
Data lakes typically employ a flat architecture, where data is stored in a distributed file system like Apache Hadoop Distributed File System (HDFS) or Amazon S3. This architecture allows for scalable storage and processing of big data. Researchers can use SQL-based querying tools, such as Apache Hive or Presto, to query and analyze data stored in the data lake. These tools provide a familiar interface for researchers who are accustomed to working with relational databases.
In addition to SQL-based querying, data lakes also support big data processing tools like Apache Spark and Hadoop MapReduce. These tools enable researchers to perform complex data transformations, machine learning, and statistical analysis on large datasets. Researchers can leverage these tools to uncover insights and patterns in clinical data that may not be apparent through traditional analysis methods.
4. Facilitating Collaboration and Reducing Data Silos
Data silos pose a significant challenge in clinical research workflows, hindering collaboration and slowing down the pace of discovery. When data is scattered across multiple systems and departments, researchers struggle to access and integrate the information they need, leading to duplication of efforts and missed opportunities for insights. This is where data lakes come into play, offering a centralized repository that promotes collaboration among researchers. By storing data from various sources in a single location, data lakes enable cross-functional teams to work together seamlessly, regardless of their specific roles or expertise. Researchers can easily access and analyze data from different domains, such as genomics, electronic health records, and clinical trial results, fostering a more holistic approach to research. Moreover, data lakes eliminate the need for time-consuming data retrieval and transformation processes, allowing researchers to focus on what they do best: making groundbreaking discoveries. Case studies have demonstrated the tangible benefits of collaboration through data lakes in clinical research. For example, a leading pharmaceutical company implemented a data lake to facilitate collaboration between its research and development teams, resulting in a 30% reduction in drug development timelines and a 20% increase in the number of successful clinical trials. By breaking down data silos and enabling seamless collaboration, data lakes are revolutionizing the way clinical research is conducted, ultimately accelerating the development of life-saving treatments and improving patient outcomes.
5. Scalable and Flexible Foundation for Advanced Analytics and Machine Learning
Data lakes provide a powerful and adaptable foundation for advanced analytics and machine learning in clinical research. These technologies play a crucial role in extracting valuable insights from vast amounts of data, enabling researchers to make data-driven decisions and accelerate the discovery of new treatments and therapies. By storing and processing large volumes of structured and unstructured data, data lakes offer the scalability and flexibility needed to support complex analytics and machine learning workflows.
One of the key advantages of data lakes is their ability to store and process diverse types of data, including electronic health records, imaging data, genomic data, and sensor data from wearable devices. This heterogeneous data can be easily ingested, stored, and analyzed within a data lake, providing a comprehensive view of patient health and enabling researchers to identify patterns and correlations that may not be apparent from individual data sources alone.
Data lakes also enable real-time analytics and machine learning pipelines, allowing researchers to quickly process and analyze incoming data streams. This is particularly important in clinical research, where timely insights can inform critical decisions and improve patient outcomes. With data lakes, researchers can develop and deploy machine learning models that continuously learn and adapt as new data becomes available, enabling more accurate predictions and personalized treatment recommendations.
Some examples of advanced analytics and machine learning applications in clinical research using data lakes include:
- Predictive modeling to identify patients at high risk of developing certain conditions or complications
- Natural language processing to extract insights from unstructured clinical notes and patient-reported outcomes
- Image analysis to identify biomarkers and predict treatment response from medical imaging data
- Genomic analysis to identify genetic variants associated with disease risk and drug response
By leveraging the power of data lakes, clinical researchers can unlock the full potential of their data and drive innovation in healthcare delivery and personalized medicine.
6. Accelerating Research Workflows and Time-to-Insight
Data lakes play a crucial role in streamlining clinical research workflows and reducing time-to-insight. By centralizing and harmonizing data from various sources, such as electronic health records, clinical trials, and real-world evidence, data lakes enable researchers to access and analyze vast amounts of information quickly and efficiently. This centralized data management approach eliminates the need for time-consuming data silos and manual data integration processes, allowing researchers to focus on extracting valuable insights from the data. Data lakes also facilitate faster hypothesis testing and iteration by providing a flexible and scalable platform for data exploration and analysis. Researchers can easily combine different data sets, apply advanced analytics techniques, and visualize results to identify patterns, trends, and correlations that may lead to new discoveries or improved patient outcomes. Real-world examples demonstrate the impact of data lakes on accelerating research workflows. For instance, a leading pharmaceutical company leveraged a data lake to integrate clinical trial data with real-world evidence, enabling them to identify potential drug repurposing opportunities and optimize clinical trial design. Another example involves a healthcare organization that used a data lake to analyze patient data across multiple therapeutic areas, resulting in the identification of novel biomarkers and the development of personalized treatment strategies. By harnessing the power of data lakes, clinical researchers can significantly reduce the time required to generate actionable insights, ultimately accelerating the pace of scientific discovery and improving patient care.
7. Challenges and Considerations
While data lakes offer immense potential for streamlining clinical research workflows, several challenges and considerations must be addressed to ensure their effective implementation and utilization. Data governance and security are critical aspects of data lake management, as the centralized storage of sensitive clinical data necessitates robust access controls, encryption, and compliance with regulatory requirements such as HIPAA and GDPR. Data quality and standardization also pose significant challenges, as data from diverse sources may have inconsistent formats, missing values, or errors that need to be identified and rectified before the data can be reliably analyzed. Integrating data lakes with existing clinical research infrastructure, such as electronic health record systems, clinical trial management systems, and data visualization tools, requires careful planning and interoperability considerations to ensure seamless data flow and compatibility. Moreover, leveraging data lakes effectively for clinical research demands a unique set of skills, including expertise in data engineering, data science, and domain knowledge in clinical research. Organizations must invest in building and nurturing teams with the necessary skill sets to design, implement, and maintain data lakes, as well as to derive actionable insights from the vast amounts of data stored within them.
8. Conclusion
Data lakes have emerged as a powerful tool for clinical research organizations, offering numerous benefits that streamline workflows and enhance decision-making. By providing a centralized repository for structured and unstructured data, data lakes enable researchers to access and analyze vast amounts of information quickly and efficiently. The ability to integrate data from multiple sources, including electronic health records, clinical trial management systems, and wearable devices, allows for a more comprehensive view of patient data and facilitates the identification of patterns and insights that may not have been apparent otherwise. Moreover, data lakes support advanced analytics techniques, such as machine learning and natural language processing, which can help accelerate the drug discovery and development process. As the volume and complexity of healthcare data continue to grow, the role of data lakes in clinical research is expected to become increasingly critical. Organizations that invest in data lake technology will be well-positioned to harness the power of big data and drive innovation in the field. To successfully implement a data lake for clinical research, it is recommended that organizations develop a clear data governance framework, ensure data security and privacy, and foster a culture of collaboration and data-driven decision-making. By doing so, they can unlock the full potential of their data assets and revolutionize the way clinical research is conducted.